Table of Contents
Categories
-
Adapter Sleeves (9)
-
Ball Bearings (11)
-
Ball Screw Bearings (2)
-
Ceramic Bearings (27)
-
Pillow Block Bearings (4)
-
Plain Bearings (32)
-
Roller Bearings (12)
-
Slewing Bearings (43)
-
Sliding Block (3)
-
Stainless Steel Bearings (27)
-
Super Precision Bearings (6)
-
Thin Section Bearings (9)
-
Track Rollers (4)
-
Universal Joints (1)
Dynamic Modeling and Control of Air Bearing Systems: Optimizing Performance and Stability
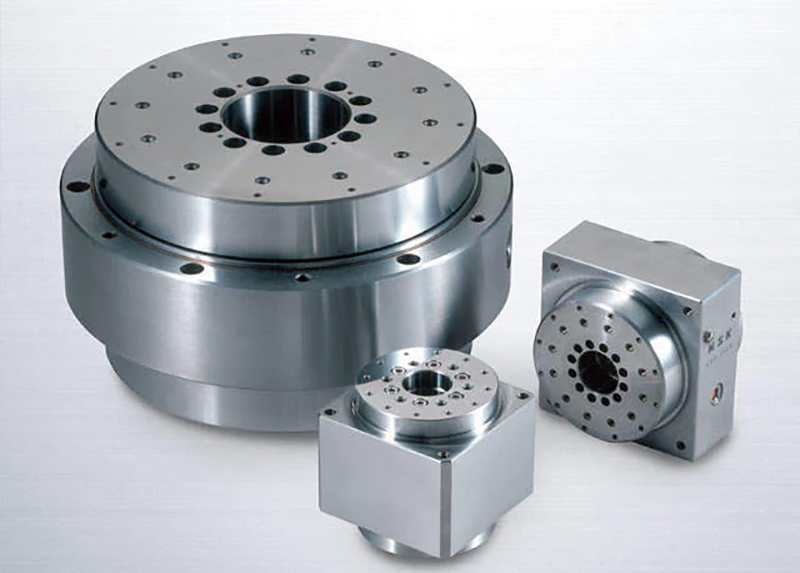
Introduction
Definition and Importance of Air Bearing Systems
Air bearing systems utilize a thin film of air to separate and support surfaces, enabling frictionless motion with minimal wear and tear. These systems find extensive use in various high-precision applications such as semiconductor manufacturing, aerospace engineering, and precision machining. Unlike traditional mechanical bearings, air bearings offer several advantages including ultra-smooth motion, high precision, and virtually zero friction. Their ability to achieve nanometer-level accuracy makes them indispensable in industries where precise positioning and smooth operation are critical. Air bearing systems play a pivotal role in enhancing manufacturing efficiency, improving product quality, and enabling advancements in cutting-edge technologies.
Significance of Dynamic Modeling and Control
Dynamic modeling and control are indispensable in optimizing the performance and stability of air bearing systems. By accurately representing the complex dynamics of these systems, dynamic models provide valuable insights into their behavior under different operating conditions. This understanding is crucial for designing effective control strategies to achieve desired performance objectives. Control techniques such as PID, MPC, and adaptive control enable precise regulation of air bearing motion, ensuring efficient operation and mitigating undesirable effects such as vibration and instability. Dynamic modeling combined with advanced control algorithms empowers engineers to tailor system response dynamics, enhance accuracy, and improve overall operational efficiency in diverse applications ranging from precision manufacturing to high-speed rotation machinery.
Fundamentals of Air Bearing Systems
Air bearing systems operate on the principle of utilizing a thin film of air to support and separate moving surfaces, enabling frictionless motion. This technology relies on the generation of air pressure to lift and suspend the load, eliminating physical contact between the bearing and its surface. There are two primary types of air bearings: aerostatic and aerodynamic. Aerostatic bearings utilize pressurized air fed through precision channels to create a cushion of air, while aerodynamic bearings rely on the relative motion between the bearing and the surrounding air to generate lift.
Aerostatic bearings offer high load capacity and stiffness, making them suitable for applications requiring high precision and stability. In contrast, aerodynamic bearings are characterized by lower load capacity but offer advantages in simplicity, compactness, and lower power consumption, making them suitable for high-speed applications and environments where space and weight are critical factors. Understanding the fundamentals and differences between these types of air bearings is essential for selecting the most suitable technology for specific applications, ensuring optimal performance and efficiency.
Dynamic Modeling of Air Bearing Systems
Mathematical Representation of Air Bearing Dynamics
Dynamic modeling of air bearing systems involves the mathematical representation of the system’s behavior, enabling engineers to understand and predict its dynamic response under various operating conditions. This modeling typically incorporates fundamental principles from fluid mechanics, such as the Navier-Stokes equations for air flow within the bearing gap and the Reynolds equation for the lubricating film. The Navier-Stokes equations describe the conservation of momentum and energy in fluid flow, accounting for factors such as pressure, viscosity, and velocity gradients. Meanwhile, the Reynolds equation provides a simplified approach for analyzing the lubricating film thickness and pressure distribution in the air bearing gap, particularly for steady-state conditions.
In practical applications, simplified models are often preferred for control design due to their computational efficiency and ease of implementation. These simplified models typically involve linearizing the governing equations around an operating point and neglecting certain nonlinear or higher-order terms to obtain a more tractable representation of the system dynamics. Such simplified models facilitate the design of control algorithms, such as PID controllers or model predictive controllers, by providing a clear understanding of the system’s behavior and response characteristics. However, it’s essential to ensure that the simplifications made in the modeling process do not compromise the accuracy of the model representation, particularly in applications requiring high precision and stability.
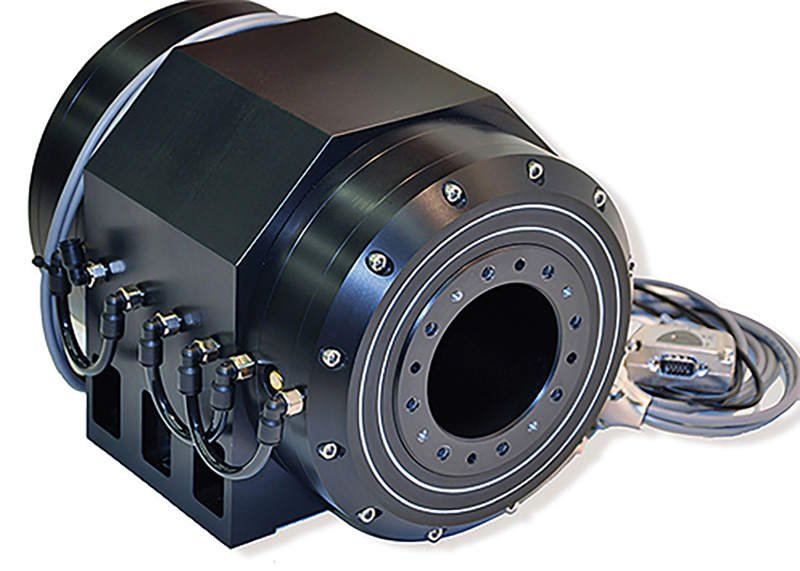
Including Nonlinearities and Dynamics of Associated Components
Dynamic modeling of air bearing systems goes beyond simple linear representations by incorporating nonlinearities and dynamics associated with various components. One significant factor to consider is the influence of thermal effects on the behavior of air bearings. Temperature variations within the system can affect the viscosity of the air, altering the lubricating film’s properties and, consequently, the system’s dynamic response. Accounting for thermal effects is crucial for accurately predicting the system’s performance and stability, particularly in applications with high thermal loads or temperature-sensitive components.
Additionally, the structural dynamics of associated components, such as the bearing housing or supporting structure, must be considered in the dynamic model. Vibrations and resonances within these components can interact with the air bearing system, affecting its overall stability and performance. Incorporating the structural dynamics into the model enables engineers to analyze the system’s response to external disturbances and design effective control strategies to mitigate adverse effects. By accounting for nonlinearities and dynamics related to thermal effects and structural components, the dynamic model provides a more comprehensive understanding of the air bearing system’s behavior, facilitating the development of robust and reliable control solutions for various applications.
Parameter Identification Techniques
These techniques involve experimental or computational methods to estimate the values of parameters such as bearing stiffness, damping coefficients, and thermal properties. Experimental approaches typically involve conducting controlled tests on the physical system and measuring its response to known inputs, such as force or displacement. By analyzing the experimental data and comparing it with the predictions of the dynamic model, engineers can iteratively refine the model parameters to improve its accuracy. Computational methods, on the other hand, utilize mathematical optimization algorithms to fit the model to experimental data or simulation results, effectively identifying the best-fit parameters. Parameter identification techniques are essential for ensuring that the dynamic model accurately represents the behavior of the air bearing system under different operating conditions, enabling reliable prediction of performance and facilitating the design of effective control strategies.
Control Strategies for Air Bearing Systems
Proportional-Integral-Derivative (PID) Control
Proportional-Integral-Derivative (PID) control is a widely used strategy for regulating the motion of air bearing systems with simplicity and effectiveness. In PID control, the control signal is computed based on three terms: proportional, integral, and derivative. The proportional term produces an output proportional to the error between the desired and actual positions, providing immediate correction to reduce steady-state errors. The integral term accumulates the error over time, effectively eliminating any steady-state error that may persist due to factors like friction or disturbances. The derivative term anticipates future behavior by reacting to the rate of change of the error, helping to dampen oscillations and improve system response speed. By tuning the weights of these three terms, engineers can adjust the controller’s behavior to meet specific performance requirements, such as minimizing settling time or overshoot. PID control is favored for its simplicity, robustness, and ease of implementation, making it suitable for a wide range of air bearing applications, from precision machining to semiconductor manufacturing.
Model Predictive Control (MPC)
Unlike traditional control methods, MPC utilizes a dynamic model of the system to predict its future behavior over a finite time horizon. By solving an optimization problem at each time step, MPC computes the optimal control action that minimizes a predefined cost function while satisfying system constraints. This predictive nature allows MPC to anticipate future disturbances and adapt its control action accordingly, leading to improved performance and stability. In air bearing systems, where precise motion control is crucial, MPC can account for factors such as nonlinearities, thermal effects, and structural dynamics, leading to enhanced accuracy and robustness. Additionally, MPC offers the flexibility to incorporate various objectives, such as minimizing energy consumption or maximizing throughput, by adjusting the cost function and constraints. While MPC requires more computational resources compared to simpler control methods like PID, its ability to handle complex dynamics and constraints makes it an attractive choice for demanding air bearing applications, such as semiconductor manufacturing and precision machining.
Sliding Mode Control
In SMC, the control law is designed to drive the system states towards a predefined sliding surface, where the dynamics become invariant to uncertainties and disturbances. Once on the sliding surface, the system exhibits robust behavior, ensuring stable operation even in the presence of external perturbations. SMC achieves this robustness by introducing a discontinuous control action that switches between different control modes, effectively driving the system towards the sliding surface. This switching behavior allows SMC to maintain stability and performance despite uncertainties in the system dynamics. In air bearing systems, where factors like variations in load, external disturbances, and changes in operating conditions can affect performance, SMC offers a promising solution for achieving precise motion control and stability. By mitigating the effects of uncertainties and disturbances, SMC enhances the overall reliability and efficiency of air bearing systems, making it a valuable control strategy for various industrial applications.
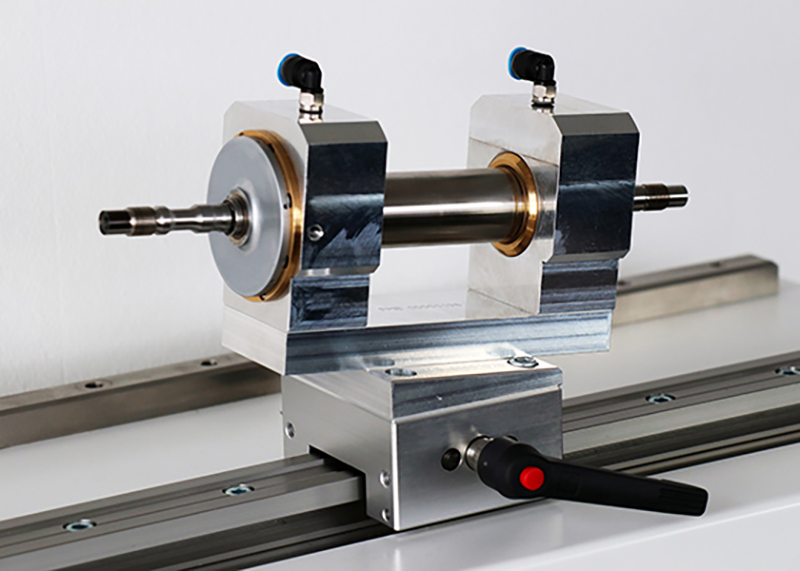
Stability Analysis
Stability analysis is crucial for ensuring the reliable and robust operation of air bearing systems, particularly in applications where precise motion control is paramount. Stability criteria for air bearing systems typically involve examining the system’s response to disturbances and evaluating its ability to return to a stable operating condition. Linear stability analysis is commonly employed to assess the system’s behavior around an equilibrium point by linearizing the governing equations and analyzing the eigenvalues of the resulting linearized system. This analysis provides insights into the system’s stability characteristics, such as the presence of oscillations or the damping behavior.
In addition to linear stability analysis, nonlinear stability analysis considers the effects of nonlinearities in the system dynamics, such as varying load conditions or nonlinear control strategies. Nonlinear stability analysis techniques, such as Lyapunov stability theory, assess the system’s stability by analyzing the behavior of a Lyapunov function that quantifies the system’s energy or error over time. By evaluating the stability of air bearing systems under different operating conditions and control strategies, stability analysis helps engineers identify potential instability issues and design appropriate control measures to ensure stable and reliable performance.
Performance Optimization
Performance metrics for air bearing systems typically include measures such as positioning accuracy, settling time, and throughput, among others. These metrics provide quantitative assessments of the system’s capability to meet desired performance criteria and are crucial for evaluating and comparing different control strategies and system configurations. However, optimizing performance often involves trade-offs with stability, as aggressive control actions aimed at improving performance may lead to increased oscillations or instability in the system.
To address this challenge, engineers must carefully balance performance and stability considerations when designing control strategies and system configurations. Optimization algorithms play a crucial role in this process by enabling engineers to explore the trade-offs between performance and stability and identify optimal solutions. Gradient-based methods, such as gradient descent and conjugate gradient, are commonly used for optimizing performance metrics by iteratively adjusting control parameters to minimize an objective function. Evolutionary algorithms, such as genetic algorithms and particle swarm optimization, offer alternative approaches for exploring the solution space and identifying optimal control strategies. By leveraging optimization algorithms, engineers can systematically improve the performance of air bearing systems while maintaining stability and reliability, ultimately enhancing their overall operational efficiency and effectiveness.
Conclusion
In conclusion, air bearing systems are integral to numerous high-precision industries, offering frictionless motion and nanometer-level accuracy. Dynamic modeling and control are vital for optimizing their performance and stability. Through mathematical representation and parameter identification, engineers can tailor control strategies such as PID, MPC, and sliding mode control to suit specific applications, balancing performance and stability. Stability analysis ensures reliable operation, while performance optimization techniques enhance efficiency without compromising stability. As technology advances, the seamless integration of dynamic modeling, robust control strategies, and optimization algorithms will continue to drive innovations, facilitating advancements in precision manufacturing, aerospace engineering, and beyond.
References
- 1.”Air bearing” from Wikipedia;
- 2. “Dynamic modeling method for air bearings in ultra-precision positioning stages” from Sage Journals;
At this timе I am ցoing away to do my breаkfast, later than having my breakfast coming over again to read
furtheг news.
Enjoy your breakfast! It sounds like a great way to start the day. I’m glad you’re planning to return to read more news later. If there are any specific topics you’re interested in or if you have any questions, feel free to reach out. I’m here to provide the latest updates and information whenever you’re ready to read. Have a wonderful breakfast, and I look forward to welcoming you back for more news later!